
Introducing RL Unplugged: Benchmarks for Offline Reinforcement Learning
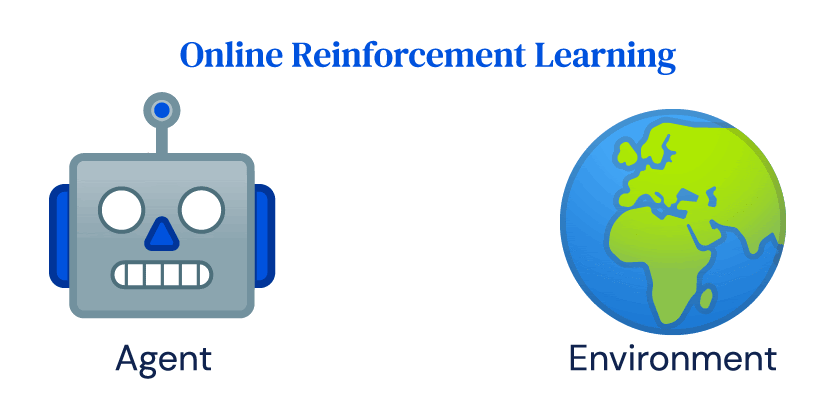
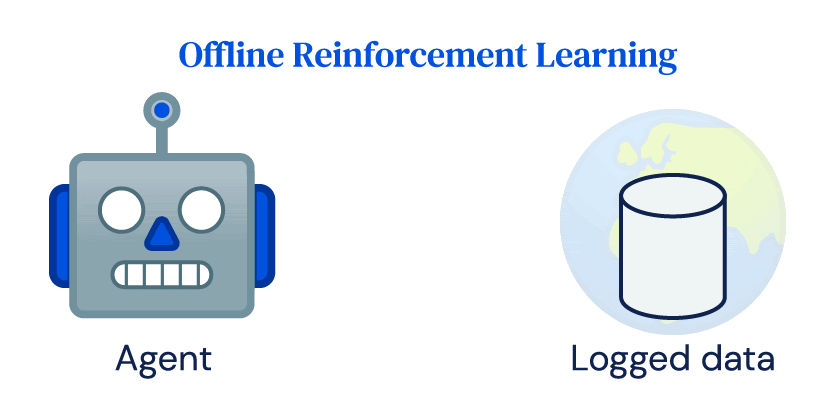
In the field of artificial intelligence (AI), offline reinforcement learning (RL) has shown promise in learning policies directly from logged data instead of relying on real-time interactions with the environment. However, the adoption of RL for real-world applications has been limited due to the challenges posed by expensive and potentially dangerous systems such as power plants, robots, healthcare systems, and self-driving cars. These systems are not easily compatible with the exploration aspect of RL and the data requirements of online RL algorithms.
While offline RL methods have demonstrated positive results in well-known benchmark domains, the lack of standardized evaluation protocols, varying datasets, and absence of baselines make it difficult to compare algorithms. Additionally, the current offline RL literature lacks representation of important properties found in potential real-world application domains, including partial observability, high-dimensional sensory streams (e.g., images), diverse action spaces, exploration problems, non-stationarity, and stochasticity.
In order to address these challenges and promote reproducibility and accessibility in offline RL research, we present RL Unplugged, a novel collection of task domains, associated datasets, and a clear evaluation protocol. Our benchmark suite includes popular domains such as the DM Control Suite and Atari 2600 games, as well as more challenging environments like the real-world RL (RWRL) suite tasks and DM Locomotion tasks. By standardizing the environments, datasets, and evaluation protocols, we aim to facilitate advancements in offline RL.
The Four Key Contributions of RL Unplugged
- Unified API for Datasets: We provide a unified API for accessing datasets, ensuring consistency and ease of use for researchers.
- Variety of Environments: Our benchmark suite offers a diverse set of environments, encompassing both popular domains and more challenging tasks. This allows researchers to evaluate their algorithms in various scenarios.
- Clear Evaluation Protocols: We introduce clear evaluation protocols that enable standardized comparisons between different offline RL algorithms. This promotes fair assessments and facilitates progress in the field.
- Reference Performance Baselines: We establish reference performance baselines for the RL Unplugged benchmarks, aiding researchers in understanding the strengths and weaknesses of their algorithms.