
Measuring Progress in AI: Introducing the Standardised Test Suite (STS)
Interacting effectively with humans is a crucial skill for AI agents. However, measuring progress in human interaction is a complex task. In this article, we explore a new method called the Standardised Test Suite (STS) that evaluates agents in multi-modal interactions.
The Importance of Measuring Progress
Without a way to measure progress, training AI agents to interact with humans becomes challenging. The STS methodology addresses this issue by placing agents in real human scenarios, allowing them to engage in tasks and answer questions within a 3D simulated environment.
How STS Works
The STS methodology involves replaying scenarios that have been extracted from real human interactions. Agents are then given instructions and have control to complete the interaction offline. These agent continuations are recorded and sent to human raters who determine whether the interaction was successful or not. Agents are ranked based on their success rate across different scenarios.
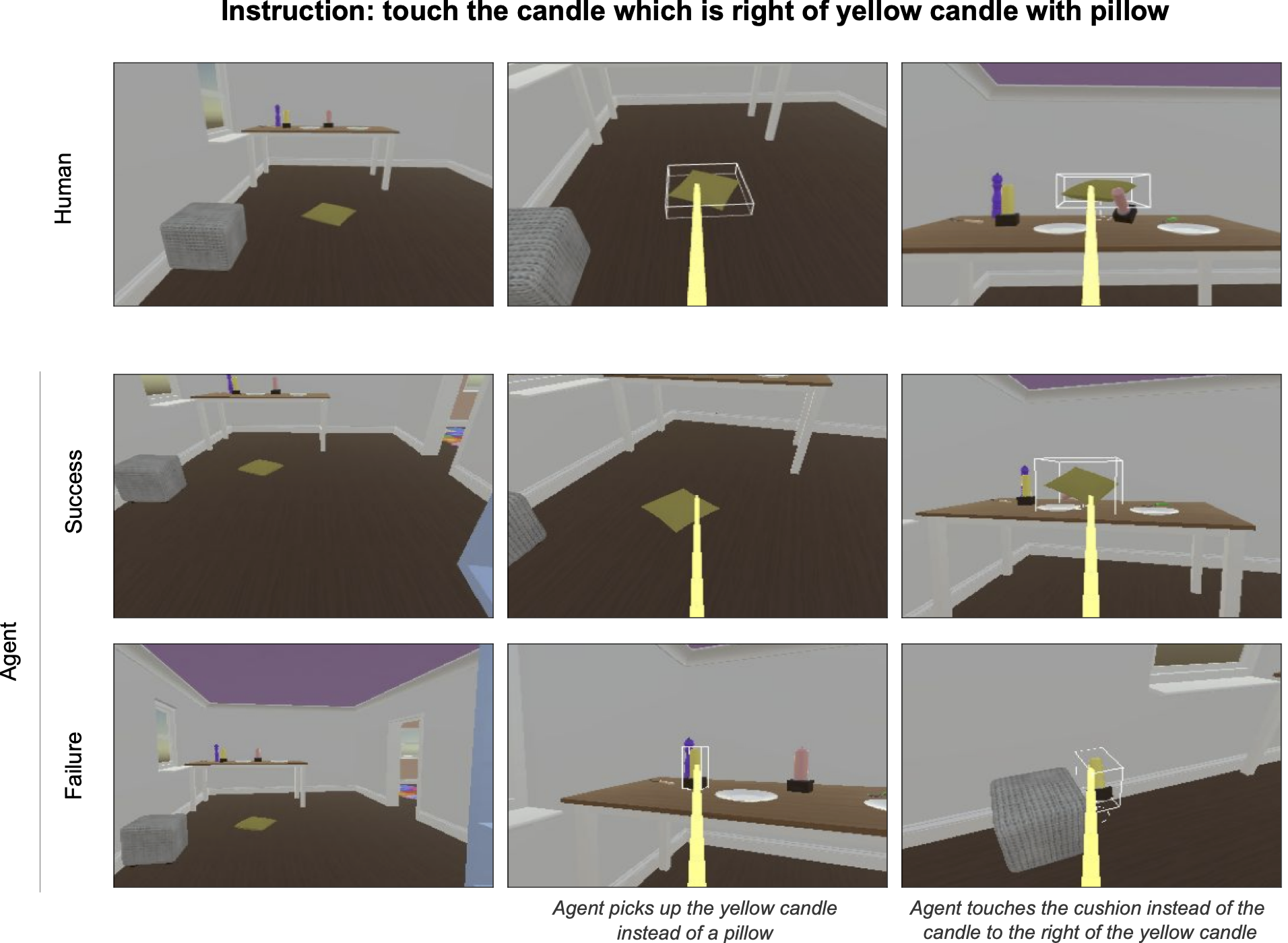
Challenges of Training Agents for Human Interactions
Teaching AI agents to interact fluidly with humans is difficult because many human behaviors cannot be easily codified. While reinforcement learning has been successful in solving games, it falls short when it comes to human interactions. The complexity of understanding context, nuances, and subjective factors makes it challenging to train agents effectively.
The Limitations of Traditional Evaluation Methods
Interactive evaluation by humans is costly and time-consuming, and it’s challenging to control the exact instructions given to agents. Previous evaluation methods, such as losses and scripted probe tasks, don’t align well with interactive evaluation. The STS offers a more controlled and faster evaluation metric that closely reflects the goal of creating AI agents that interact well with humans.
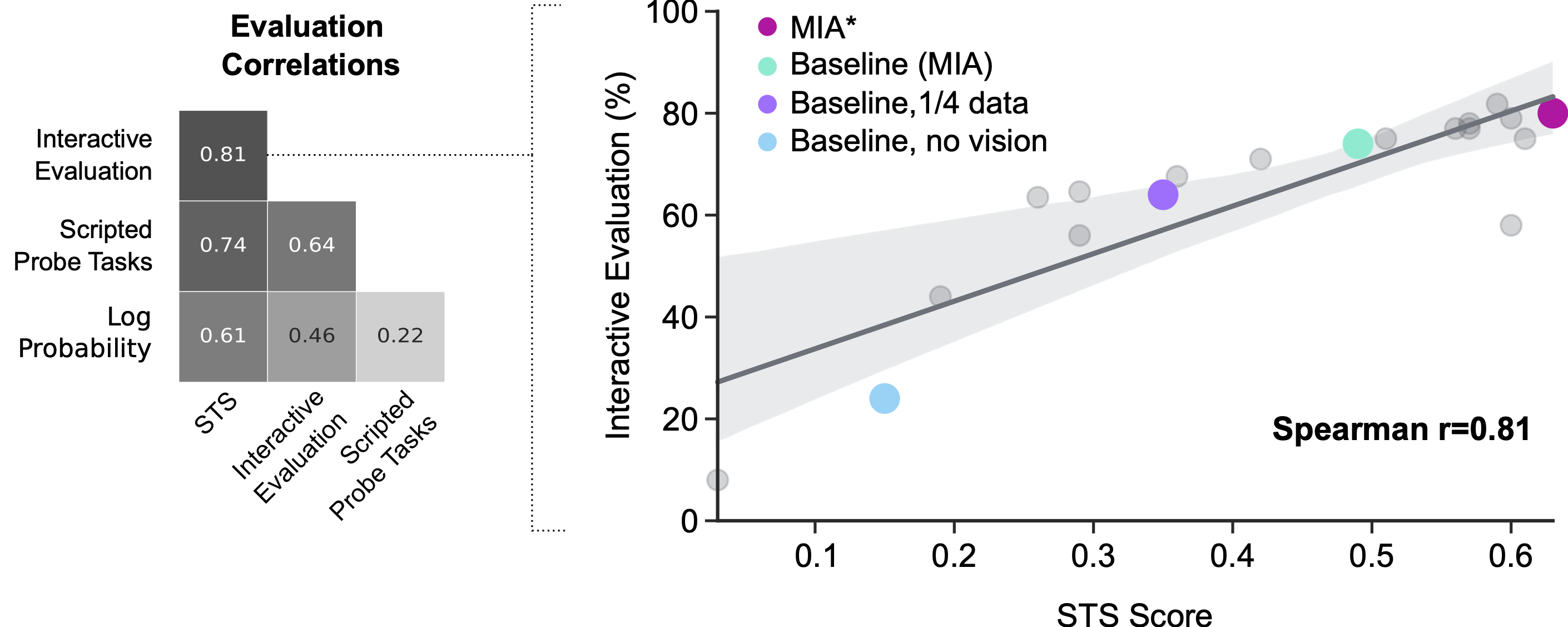
Advancing the Field of Human-Agent Interaction Research
The STS methodology aims to provide a cost-effective way to train and evaluate AI agents for human interaction. While human annotation is still required, there is potential for automation of this process, which would significantly speed up evaluation. We encourage researchers to adopt the STS methodology to accelerate progress in this field and eventually create AI agents that excel in human interactions.