
Building a responsible approach to data collection with the Partnership on AI
DeepMind strives to uphold the highest standards of safety and ethics in everything it does, as outlined in its Operating Principles. An essential aspect of this is how data is collected. Over the past year, DeepMind has collaborated with the Partnership on AI (PAI) to tackle the challenges associated with responsible human data collection and has developed standardized best practices and processes.
Human data collection
To ensure the well-being and rights of human participants involved in studies, DeepMind established the Human Behavioural Research Ethics Committee (HuBREC) three years ago. This committee, similar to academic institutional review boards (IRBs), oversees behavioral research that involves studying how humans interact with AI systems in decision-making processes.
In addition to behavioral research, the AI community has increasingly engaged in “data enrichment” tasks, such as data labeling and model evaluation, which involve paying individuals to improve AI models. These tasks often lack proper governance systems and raise ethical concerns regarding worker pay and welfare. As AI models become more sophisticated, the reliance on data enrichment practices is expected to grow, necessitating stronger guidance.
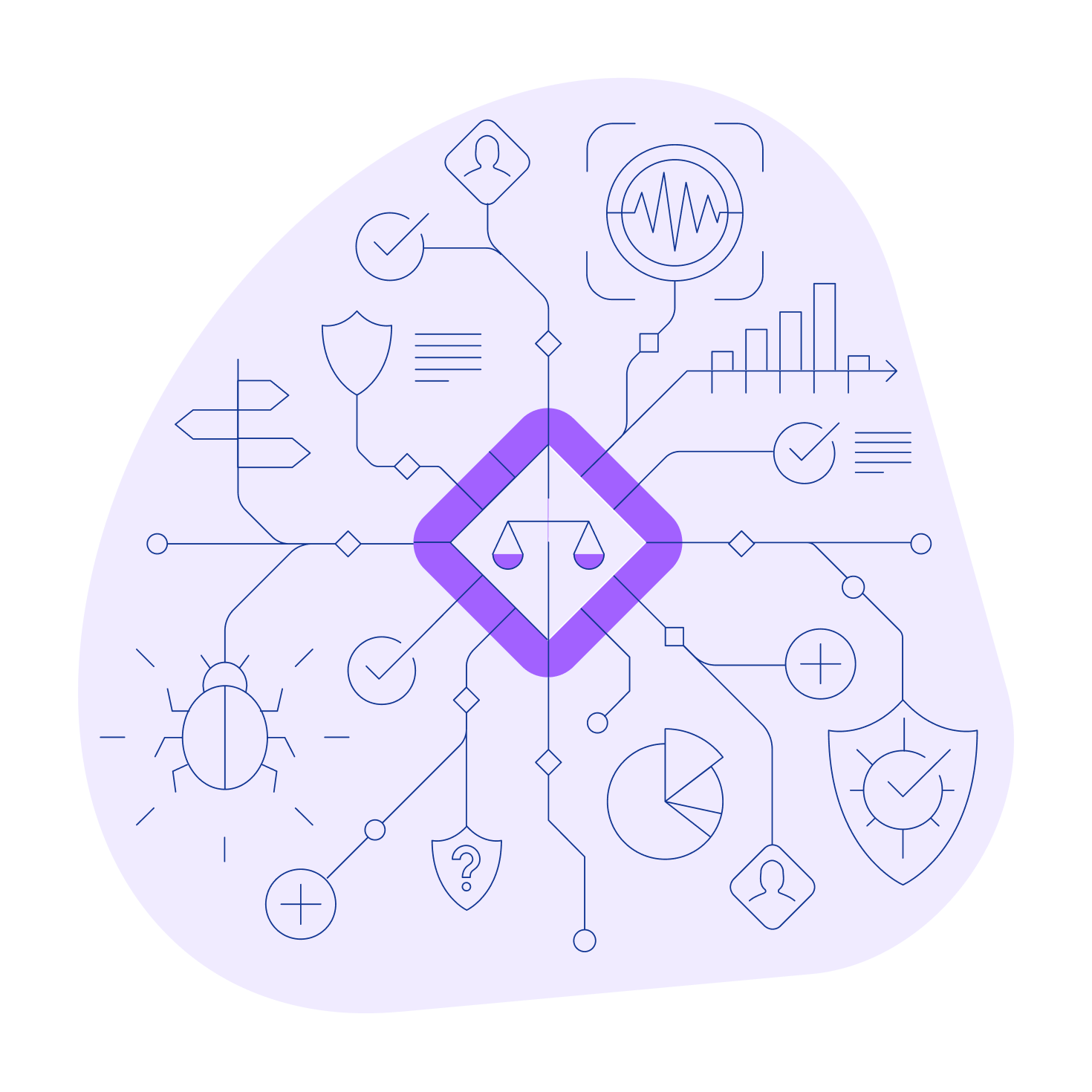
DeepMind’s commitment to AI safety and ethics includes contributing to best practices, fairness, and privacy to avoid unintended harmful outcomes, as per its Operating Principles.
The best practices for data enrichment
In collaboration with PAI, DeepMind developed best practices and processes for data enrichment based on the guidelines provided in PAI’s recent white paper on Responsible Sourcing of Data Enrichment Services. These include:
- Selecting an appropriate payment model that ensures all workers are paid above the local living wage.
- Designing and running a pilot project before launching a data enrichment task.
- Identifying suitable workers for the desired task.
- Providing clear instructions and/or training materials for workers to follow.
- Establishing effective and regular communication channels with workers.
DeepMind and PAI collaborated in creating policies and resources, incorporating feedback from various internal teams, such as legal, data, security, ethics, and research. After piloting these practices on a small scale, they were implemented across the organization. These guidelines have improved study design and execution, expediting approval and launch processes while ensuring a better experience for individuals involved in data enrichment tasks.
For more details on responsible data enrichment practices and how DeepMind has integrated them into its existing processes, refer to PAI’s case study, Implementing Responsible Data Enrichment Practices at an AI Developer: The Example of DeepMind. PAI also offers additional resources for AI practitioners and organizations seeking to develop similar processes.
Looking forward
While these best practices form the foundation of DeepMind’s work, relying solely on them is not enough to ensure the highest standards of welfare and safety for research participants or workers. DeepMind has a dedicated human data review process that allows ongoing engagement with research teams to identify and mitigate risks on a project-by-project basis.
This work serves as a resource for other organizations interested in improving their data enrichment sourcing practices. DeepMind hopes that this collaboration leads to cross-sector discussions and the development of industry standards for responsible data collection, ultimately benefiting the AI community as a whole.
To learn more about DeepMind’s Operating Principles, visit here.